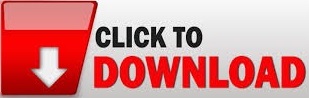
More complicated than data warehouses, data lakes typically need to be set up by data scientists or engineers with the expertise to interpret and organize raw data before it can be processed. These are cost-effective storage solutions for businesses who need to rapidly capture and retain huge amounts of data without needing much transformation. When queries are run, they can run against the limited, smaller data set with the specific tags rather than having to process all the data stored in the lake.ĭata lakes are well-suited to storing unstructured data from disparate sources and in different formats-for example, social media posts, multimedia files, log files, emails, and data from Internet of Things (IoT) devices. Data lakes use a flat architecture to store data so it is completely unstructured, retaining it in the format in which it was originally ingested.Įach data element in the lake is assigned a unique identifier and a set of extended metadata tags. What is a Data Lake?Ī data lake is a storage repository that holds a vast amount of raw data in its native format until it is needed. This article compares both storage solutions on their features and use cases to help you better understand the difference. Rather than being mutually exclusive, they’re complementary solutions that can work effectively to provide business intelligence for organizations that implement them wisely. Data lakes and data warehouses are two ways of storing data in large quantities with very different approaches, each with its own strengths and weaknesses.
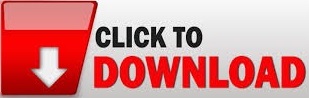